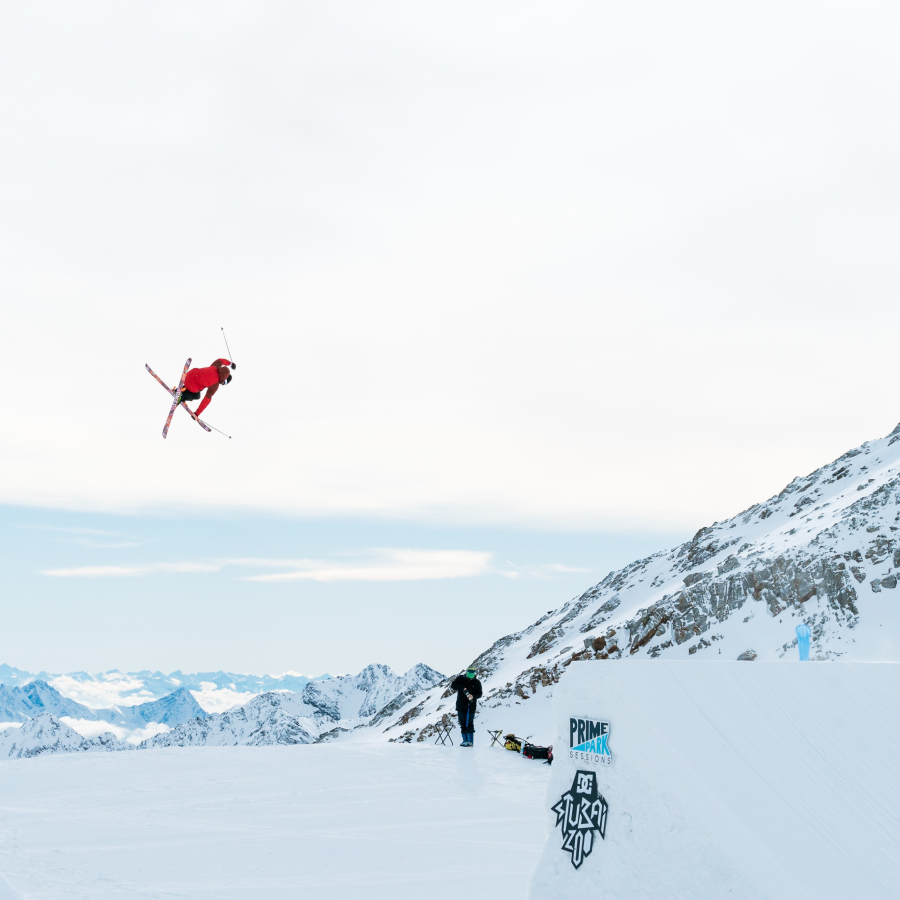
post
October 10, 2022
Combining NLP and NLU to Deliver Real ROI
There’s a common misconception in the marketplace that conversational AI basically comes down to people talking to machines. For anyone eager to capitalize on the opportunities conversational AI affords, this is a dangerous line of thinking. Part of the confusion arises from a fundamental misunderstanding of the technologies that allow machines to understand human language, whether written or spoken.
Let’s look at the fundamental pieces of technology that enable machines and humans to converse. Keep in mind, however, that these technologies are still just a small piece of the conversational AI puzzle—something we’ll get to later in this article.
There have been enormous improvements in intent recognition, entity recognition, fulfillment, voice-optimized responses, dynamic text-to-speech, machine learning, and contextual awareness that have enabled computers to both parses what people say (natural language processing, NLP) and then determine the intent behind it to reply with something useful (natural language understanding, NLU).
NLP and NLU are interconnected and can be leveraged together as a key driver toward adoption, but it can be helpful to think about them separately to form a better understanding of how they fit together.
NLP Only Gets You So Far
Many of the existing IVRs and chatbots people interact with on websites and over phones are cautionary tales of NLP working in isolation. Customers generally dread the interactions because very few questions are answered, and those answers that do come through are often missing key information. These experiences typically end with users still needing more information. It’s no surprise that Garter VP Analyst Anthony Mullen predicted back in 2021, at the Gartner Innovation & Business Solutions Summit, that “90% of the chatbots live today will be discarded by the end of 2023.”
Businesses accept sacrificing customer experience because they can still cut costs with subpar conversational experiences. Customers suffer through inept chatbots with low expectations or hit the “0” escape hatch at the first opportunity. NLP can bring users up to the point where the machine understands the goal of the user, and sublime NLP architecture can establish context by identifying specific entities or slotting things the user might have said during the utterances. But that alone isn’t enough to provide a truly worthwhile experience to users. A great NLP needs a complementary NLU that gives it direction when ambiguities arise.
NLU Completes the Circle
While the NLP’s job is to listen and form a basic understanding of what’s being said, NLU exists to provide a deep understanding of related use cases and tie in as much data about the user as possible. This is the kind of understanding people excel at.
In human-to-human conversations, we can contextualize all sorts of things right away. Even if we’re chatting with a stranger, their body language, volume, and word-choice will give us conversational guardrails in an instant. If it’s someone we’ve talked with before, we can draw on previous conversations and things we know about the person.
As the conversation develops, we can also take in contextual updates in real-time. We can link new information with existing information, isolate the meaning of requests, and factor in environmental data. If someone says, “I want to be cool.” We can take into account the comfortable air temperature in the room and remember from previous conversations that they love David Bowie to assume they mean they want to update their wardrobe, not a blast of cold air. Machines aren’t good at this kind of contextualization, and they need our help establishing it.
Along with using the best NLU technology available for any given interaction, this can be achieved through a conversational design that helps disambiguate information. There are also efforts underway to use improved learning algorithms to help machines learn more like humans, and other animals do. In the near term, human-in-the-Loop is a powerful design pattern that allows an intelligent bot to query a human when it hits a wall. Not only can the human course-correct or take over for the bot, they can also provide the bot with the contextual information it was missing so that it can evolve.
Combined Brushstrokes
There are different ways we can use NLP/NLU to create ROI. First, it’s helpful to understand that with conversational AI, NLP/NLU is just a small piece of the puzzle. It’s an important piece in that it provides users with a conversational interface that they already know how to use without training. But what’s more important is what that interface is connected to.
I’ve heard many terms used to describe this, but leveraging conversational AI requires an intelligent communication fabric. Whether you think of it as a mesh, a network, or a shared layer, this fabric exists to connect all of the people, systems, and things within your organization. With this fabric in place, you can start thinking of ways to sequence advanced disruptive technologies in ways that can provide meaningful automations. Good conversational design can help get the required information from users, connect that information with existing information, and determine which use case will lead them to a satisfying conclusion. These experiences can also go beyond the limits of talking and typing—incorporating gestures, facial expressions, visual aids, sounds, and haptic feedback.
Applications of conversational AI can take many forms. Something as sophisticated as a digital personal assistant that’s connected to every data point in your life and is always searching for ways to help you make better decisions lives at one end of the spectrum. Conversational AI can also be something less flashy, like a whisper agent that helps a human agent get through steps more quickly by providing suggested dialog and consolidating available tools based on current needs—improving both the customer and employee experience and reducing the likelihood of costly human errors. Conversational AI is also primed to go everywhere—across industries, from enterprises to restaurants.
Strive for Better Experiences
What’s critical in every use case, however, is that the people designing and evolving the bots (ideally in conjunction with the people who use them) are always looking for new ways to orchestrate technology in ways that can provide better experiences than what humans alone can provide.
Conversational AI experiences that merely match the experience a human can provide won’t provide a significant edge. Advancements in NLP/NLU have been impressive, and they continue to mount, but they still need to be placed into the broader context of an overall experience that’s better-than-human. Widespread adoption of these technologies is contingent on providing better-than-human experience (BtHX), something that few organizations in the world have accomplished.
I’ve seen firsthand: this is where the power lies. By developing a deep understanding of both customer needs and their context, you can become their new best friend. This requires an intelligent communication fabric using deep machine learning to compare situations, predict desired outcomes, and make meaningful connections between existing data and incoming data.
Imagine being greeted by an intelligent bot that is able to figure out why you’re calling without being asked. By running the number from an incoming call against recent activity organization-wide, it can determine that the call is coming from a customer who ordered something online more than a week ago that hasn’t been delivered. The bot can answer the call already knowing what you most likely need.
Behind the scenes, conversational AI relies on a variety of technologies and processes, including integrations, task automation, multichannel optimization, maintenance and optimization, and real-time analytics and reporting. The more complex a use case becomes, the less prominent NLP/NLU becomes, but when it’s leveraged properly, that’s one of its strengths. Because of the immense orchestration going on behind the scenes, it can say less while doing more and delivering real ROI.
Stay up to date
Latest Articles
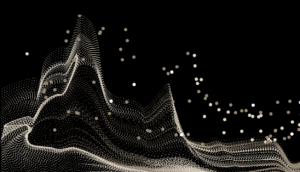
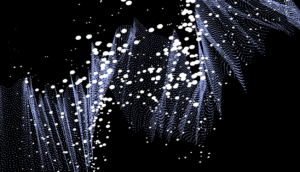
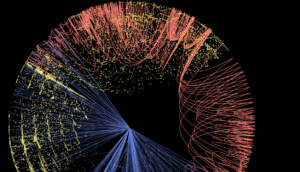