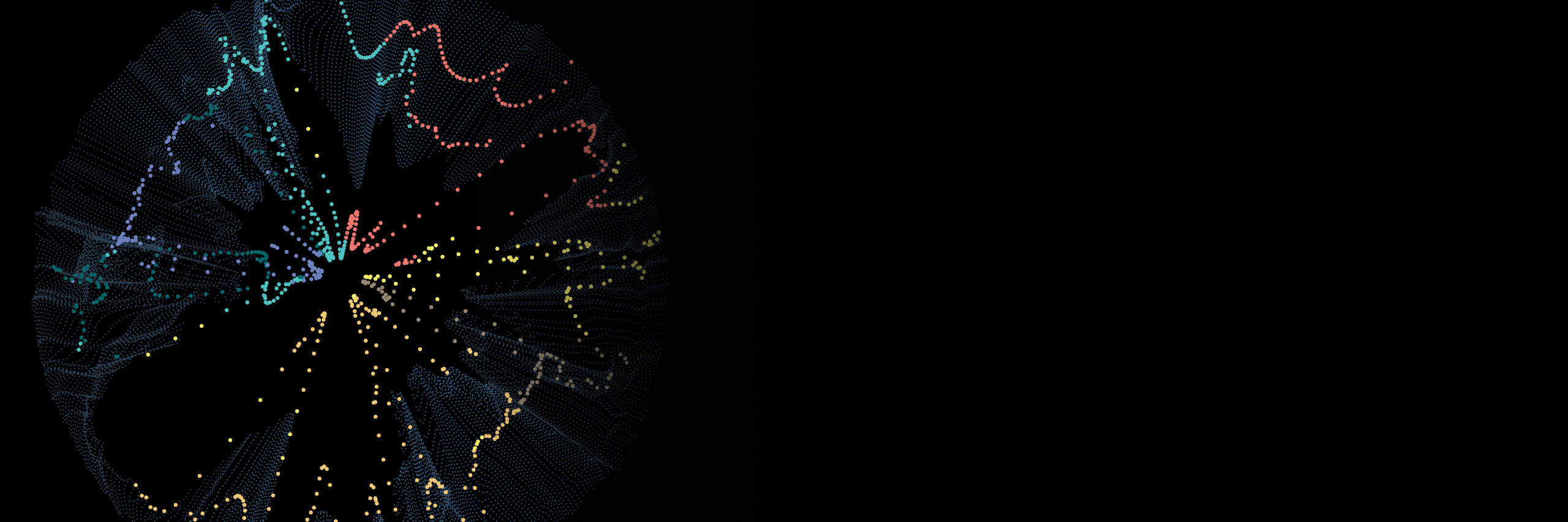
post
August 27, 2024
The Power of Retrieval-Augmented Generation in Information Management
Information overload is a major headache for organizations. The 2023 Microsoft Work Trend Index reveals that 62% of survey respondents struggle with spending too much time searching for information during their workday. Companies are inundated with mountains of reports, memos, and emails that are often disorganized and difficult to search.
Large language models (LLMs) like GPT-4 offer promising text summarization capabilities, seemingly providing a solution to this problem. However, their effectiveness is limited because they can only provide responses based on the data they’ve been trained on. Fine-tuning these models on new, company-specific information is both expensive and time-consuming, making it an impractical solution for many organizations.
Retrieval-Augmented Generation (RAG) is a revolutionary approach designed to eliminate information chaos. By combining LLMs with external knowledge bases, RAG enables organizations to obtain precise, up-to-date summaries of their information.
In this article, you’ll learn about RAG systems and how they are transforming the way organizations handle information, helping them reclaim valuable time and streamline their operations.
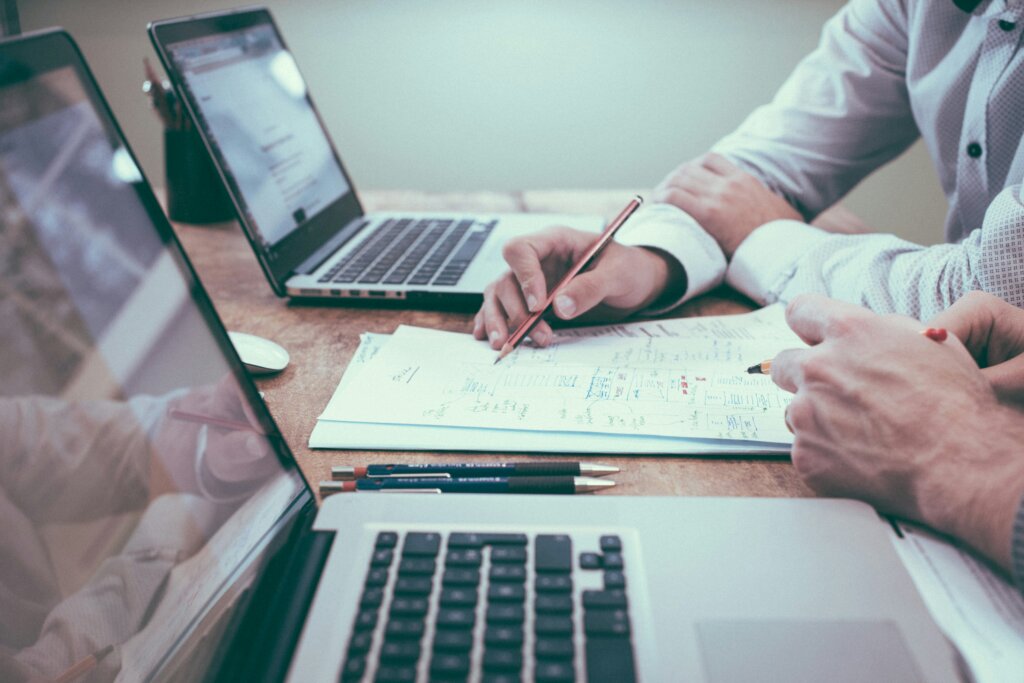
What RAG Is and How It Works
Retrieval-Augmented Generation (RAG) is a powerful tool that extends the capabilities of LLMs by giving them access to highly specific, external information. This innovative approach combines the strengths of LLMs with the precision of targeted information retrieval, making it invaluable for organizations seeking efficient knowledge management and information synthesis.
At its core, RAG pairs an LLM with an external document library. When a user poses a query, the system first searches the database for relevant information. The effectiveness of a RAG system largely depends on its search capabilities. While simple keyword searches can be limiting, advanced RAG implementations utilize semantic search. This method finds information based on the meaning and intent of the search terms, rather than just exact matches.
For instance, a query about “vacation days” would also return results containing related terms like “time off” or “annual leave”. The search results are then fed to the LLM along with the original question, allowing the model to generate a context-aware, accurate response:
“Good morning Robin—you have five days of vacation to use before the end of the year. Would you like to book some time off?”
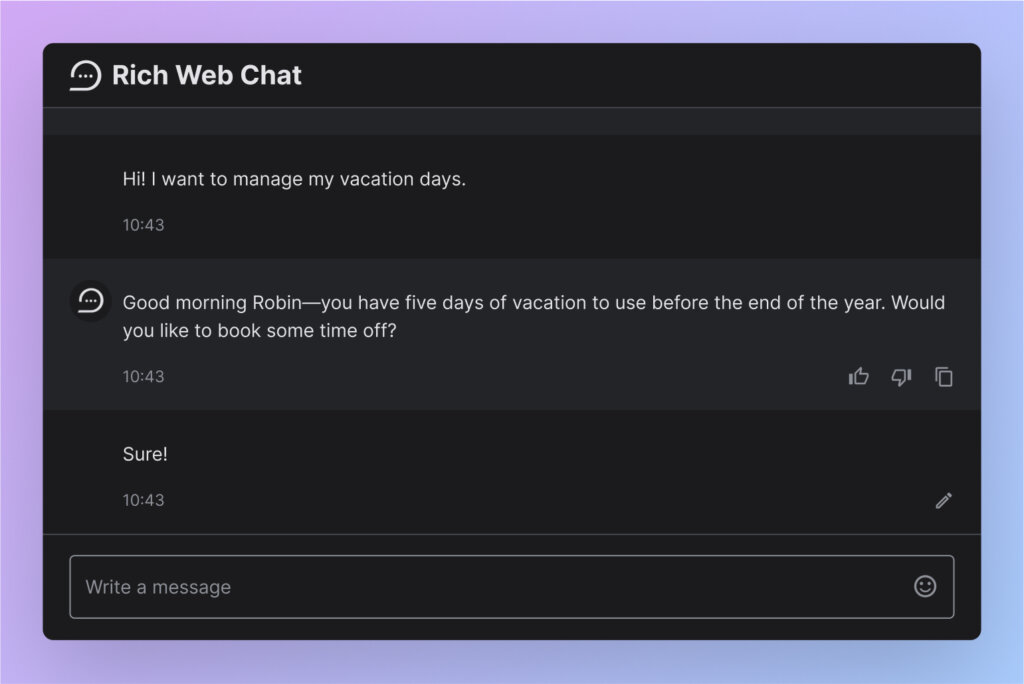
Another crucial feature of well-designed RAG systems is their ability to constrain the LLM’s responses to the information returned by the search. This significantly reduces the risk of “hallucinations”—fabricated or incorrect information that LLMs sometimes generate. If the system can’t find a suitable answer in its database, it can be directed to acknowledge this limitation rather than inventing a response.
Bringing RAG to Life in OneReach.ai’s Generative Studio X
Generative Studio X (GSX) offers a powerful tool called Lookup for building effective RAG systems that interact seamlessly with documents and data through natural language. In Lookup, users can create comprehensive document collections from a variety of sources, including PDFs, spreadsheets, and website text. When users query Lookup about their document collection, the platform employs semantic search to identify the most relevant information. This data is then summarized by an LLM and presented to the user in a chat window.
Lookup facilitates interactive and dynamic conversations with documents, allowing users to ask follow-up questions and engage deeply with their content. If the search doesn’t yield relevant information, Lookup clearly informs users that the question cannot be answered based on the available data, ensuring transparency and reliability.
Lookup integrates easily with SMS, Rich Web Chat (RWC), email, and voice conversations, offering flexible sharing options via links, QR codes, or embedded chats on websites. By utilizing a RAG system in Lookup, users can efficiently manage their document collections, set fine-tune prompts for improved information retrieval, select appropriate LLMs for summarization, and assess the relevance of responses. This approach ensures that both employees and customers have timely access to the information they need, enhancing overall efficiency and satisfaction.
Use Cases of the Lookup Tool
Lookup enables organizations to harness the power of RAG across diverse use cases. Within companies, it connects employees with essential documents and databases, facilitating processes such as onboarding, information synthesis, and discovery. Also, businesses can deploy Lookup to enhance chatbots, providing customers with real-time information on opening hours, price lists, inventory, and key contacts—up-to-date, business-specific details that LLMs alone can’t provide.
For organizations overwhelmed by documents and data, Lookup in Generative Studio X from OneReach.ai is the solution to streamline and manage your information effectively.
Want to streamline business processes and communications? Learn more about an agentic approach to workflow automation in our paper, “Using AI Agents to Establish Organizational Artificial General Intelligence (OAGI).”
Stay up to date
Latest Articles
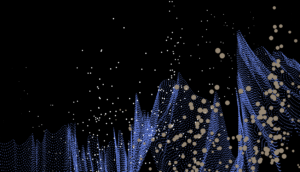
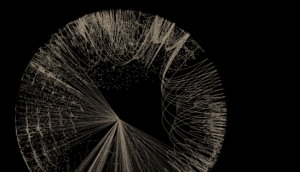
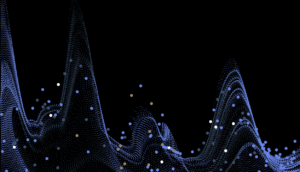