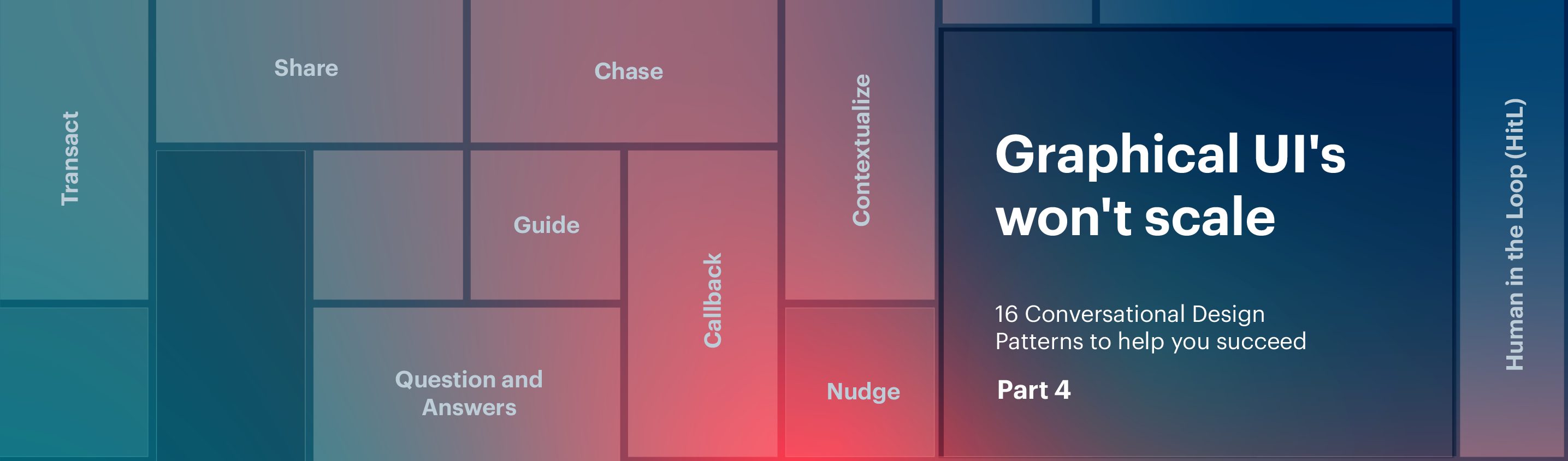
post
February 23, 2022
Conversational Design Patterns Part 4: Teach, Predict, Human-in-the-Loop and Share
Part 4: How to use conversational design patterns as collectable inspiration for your designs.
This is the last article in a four-part series sharing some of the key patterns that have emerged from successful projects. Follow us on social media (LinkedIn, Twitter, Facebook, or Instagram) to stay updated as we release new articles with the critical findings, ideas, and best practices we’re gleaning from ongoing successes and failures.
Be sure to read Part 1 , Part 2 and Part 3 of the series as well.
For decades, we’ve been studying patterns that lead to highly rated user experiences. As you read these, keep in mind that in order to succeed with conversational AI, you’ll also need the right processes, people, tools, and architecture support design that works in a coordinated way. Robb Wilson, founder of OneReach.ai, is finishing a book with resources, perspective, and practical guidance for all of aspects of the strategy required for succeeding with conversational AI. Sign up on our homepage to stay notified about Robb’s forthcoming title from Wiley, Age Of Invisible Machines.
Keeping these patterns in mind while you’re designing conversational experiences can help you create great experiences that provide efficient service for users. Let’s take a look at the last four patterns: Teach, Predict, Human in the Loop, and Share. Follow us on social media (LinkedIn, Twitter, Facebook, or Instagram) to stay tuned as we release other critical findings.
Teach
This pattern teaches users how to do something, often launching from Q&A. The purpose is to provide a series of lessons and/or instruction that can occur in a single session or across multiple sessions.
Teaching is similar to the Guide pattern we described in part two. It’s another opportunity to extend an experience over time. Your IDW can be teaching someone on a daily basis asking simple questions like “Have you practiced your Spanish?” and, depending on the response, following up with something like “If you’d like to study now, I can help you.”Your IDW ot can be trained to use different teaching styles to help different learning styles.
Share
This pattern is designed to share information with people who might need it. This proactive pattern helps disseminate information in a relevant or contextual way. Flows that use Share will send out messages or links bearing useful information.
Predict
The IDW is using past interactions and contextual data to predict what a user might be trying to do, or to suggest possible outcomes (e.g. “this option is statistically more likely to produce your desired outcome”). By looking at all the available data, Predict can be used to eliminate unnecessary steps and make conversations more efficient.
You can put the human element into your prediction algorithms and actually adjust the prediction based on the input and feedback that might have happened within the Q&A in the find. The IDW may use past interactions and some data when trying to predict what the person is likely to do.
Human-in-the-Loop (HitL)
Automations are only as good as the data at their disposal, and this powerful pattern allows humans to step in to move experiences forward and provide data to help train IDWS. People working inside the organization either feed the bot information for machine learning or script them in a particular way. There can be endless conversations or variations of conversations for humans to help with.
Solutions that incorporating this pattern can activate anyone in their organization asHuman-in-the-Loop across different channels—call centers, chat channels, text messages, or collaborative tools like Slack—to get the needed information. This gives organizations flexibility to improve experiences in real time while also updating shared library’s knowledge bases and skills. When an IDW gets stuck on a problem, it receives guidance from the appropriate human in your organization, helping it figure out what to do next or disambiguate the request.
HitL gives designers leeway if they don’t have data to train their machines. Rather than having an IDWrely solely on its programming, it can also tap into help from a human and evolve as it learns to contextualize the answers to various questions.
Stay up to date
Latest Articles
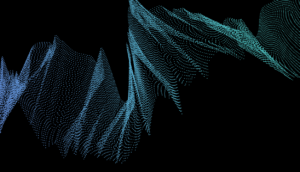
OneReach.ai Named a Leader in the IDC MarketScape for Conversational AI Software 2023
December 18, 2023